What is data mesh? How data mesh could be used in edge computing
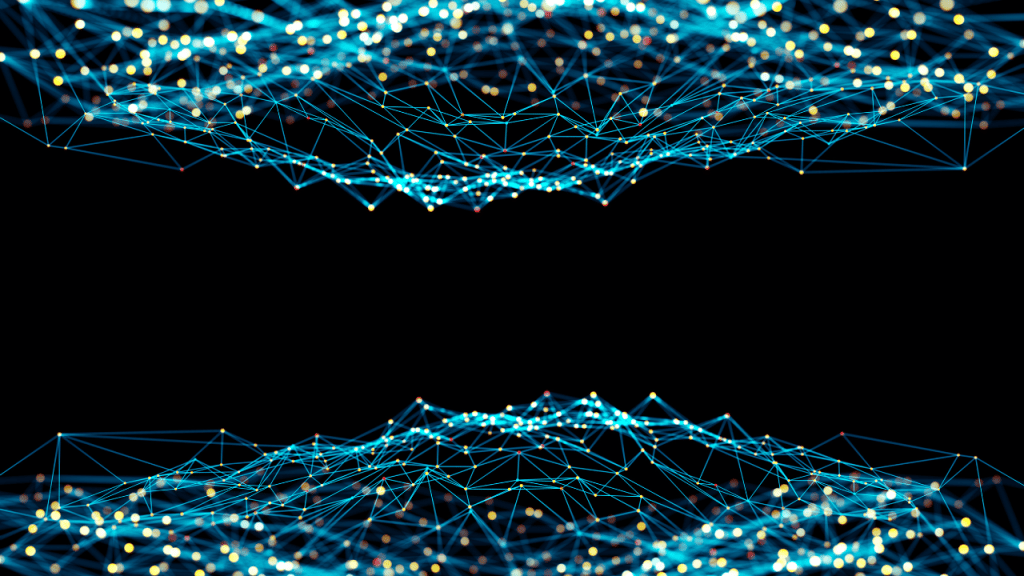
Enterprises are increasingly looking to deploy applications and services at the network’s edge to improve performance and user experience. As more data processing moves closer to the edge, it becomes essential for businesses to have a process for managing and analyzing data. One solution that has been proposed is that of a data mesh or “edge mesh.”
Should an enterprise have a data mesh in place? When should enterprises use a data mesh? What are/are there data mesh use cases in edge computing? To answer to that question, understanding what a data mesh is and how is it similar to or different from a data fabric is needed. This article answers these questions and provides insights on how a data mesh can help your business.
What is a data mesh?
Zhamak Dehghani, a principal technology consultant at Thoughtworks, first introduced data mesh to explain the new approach to designing and developing data architecture — moving from a centralized and monolithic architecture to a highly decentralized one.
Organizations often have challenges when it comes to accessing data. Data mesh aims to mitigate data availability challenges by building a connectivity layer to manage, control and support data access. By bringing together data from numerous sources across locations, a data mesh allows organizations to connect all of their data. It’s a decentralized concept that allows data to be accessible, secure, discoverable and compatible with required applications. Further, it permits firms to expand their operations faster and get more out of their data.
Data mesh has the following characteristics:
Domain-oriented decentralization: Subject-matter experts own, maintain, and develop data within their domain.
Data as a product: Teams responsible for data build and deliver data products. A data product is a self-serviceable, consumable data unit with value.
Self-serve data: Users can access the information they need without going through a centralized team.
Federated Governance: Although businesses set regulatory requirements centrally, domain teams have the freedom to apply them in ways that are appropriate for their team’s needs.
How is data mesh similar to or different from a data fabric?
A wide array of commercial, technical, and organizational settings may use data fabrics and meshes. For instance, data fabrics and data meshes can link and enhance data management software already in use. As another example, both concepts can streamline data scientist workflows by automating time-consuming, repetitive data transformation procedures and enabling self-service data access tools.
One distinction between the two concepts is that data fabric relies on metadata. In contrast, data mesh employs the knowledge of subject-matter experts who manage “domains” within the mesh. Generally, a data fabric is more technology-focused, while a data mesh is more focused on organizational transformation. A data mesh is more concerned with people and processes than architecture. In contrast, a data fabric is more of an architectural approach that solves the complex problem of data and metadata.
When should enterprises use a data mesh?
There is no one-size-fits-all answer to this question. Factors that may influence the decision to use a data mesh include:
- The size and complexity of an organization.
- The sensitivity of the data.
- The number of data sources
- The budget.
Some organizations may find that a data mesh is the best way to manage their data. In contrast, others may prefer a data fabric approach. That said, it’s crucial to remember that the two concepts aren’t mutually exclusive. Businesses may use a data mesh and a data fabric across various, or all, departments as necessary.
What are/are there data mesh use cases in edge computing?
Some common data mesh use cases in edge computing include:
IT and DevOps: A data mesh can help manage the increasing complexities of IT and DevOps. For example, it minimizes data latency by permitting direct access to query data from neighboring regions.
Marketing and Sales: A data mesh can give sales and marketing teams the ability to access customer data in real-time, regardless of location.
Machine and AI Learning Training: Intelligence and development teams can use data mesh to build virtual data catalogs and warehouses from various sources. This helps artificial intelligence (AI) and machine learning (ML) models to learn without collecting data in a single location.
Loss Prevention: In the financial sector, a data mesh implementation delivers quicker time-to-insight with less operating expenditure and operational risks. It enables international financial organizations to analyze data and spot fraud in a country or region without replicating data sets or transporting them to their central database.
Data Fabric: What is it and when should enterprises use it?
Article Topics
data fabric | data mesh | edge computing | observability | use case
Comments