What is Agentic AI?
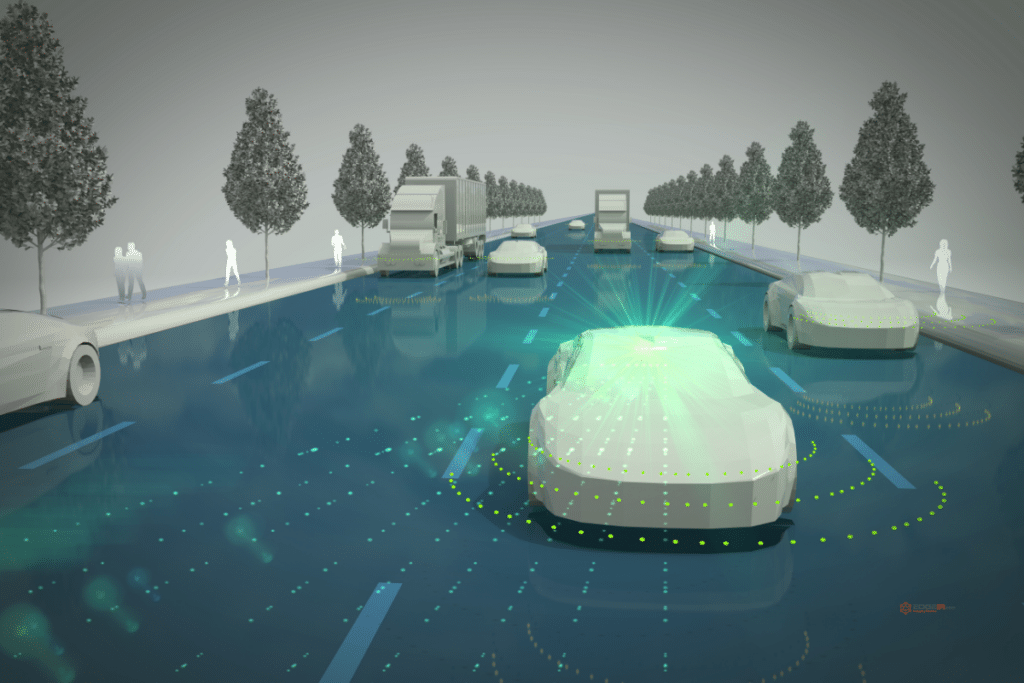
While agentic AI models can be complex, they can be optimized for efficient operation on edge devices with limited computational resources, unlike cloud servers. Before exploring their deployment on edge devices, it is essential to understand what “agentic AI models” are. The term “agentic” comes from “agency,” which refers to the capacity to act. Agentic AI models are AI systems designed to function as autonomous agents that can perceive their environment through sensors, make decisions, and take actions to achieve goals using actuators.
Agentic AI integrates the capabilities of large language models with traditional AI approaches, such as machine learning, to develop autonomous AI agents that can analyze data and make decisions independently of human intervention. While agentic AI can leverage the creative strengths of generative AI models, its primary focus is on decision-making that does not depend on human prompts. These AI models are specifically optimized to achieve particular objectives.
The defining characteristic of agentic AI is its probabilistic nature, which allows it to adapt to changing environments. Unlike traditional deterministic systems that adhere to fixed rules, agentic AI models rely on patterns to make decisions. This adaptability enables agentic AI to effectively automate processes where deterministic systems may struggle.
While agentic AI models can vary in size and complexity, some are particularly large and computationally demanding. To facilitate the efficient operation of these models on resource-constrained edge devices, one can employ various model optimization techniques, such as model compression and quantization, lightweight model architectures, hardware acceleration, and various edge computing strategies.
Key features of Agentic AI
- Autonomy: Agentic AI models have the capability to operate independently without the necessity for constant human oversight. This ability allows for continuous operations in remote edge environments where human supervision is often impractical.
- Proactivity: Unlike reactive systems, agentic AI can anticipate future conditions and take preemptive actions. For instance, in predictive maintenance systems, these models can identify potential failures and schedule repairs before a breakdown occurs.
- Adaptability: This characteristic relates to the AI’s ability to learn and evolve through interactions, which is crucial in dynamic environments where conditions change rapidly. For example, in customer service, agentic AI can adjust its responses based on emerging trends in customer inquiries without requiring manual updates.
- Efficiency and Scalability: By autonomously managing complex tasks, agentic AI enables faster operations and improves accuracy compared to traditional automated systems. Moreover, these models can be scaled to various modern edge devices by employing optimization strategies.
Applications of Agentic AI
Insights from Bain & Company indicate that public interest in agentic AI has surged dramatically, growing by 200 times from Q1 2022 to Q3 2024. Additionally, the number of academic research publications on agentic AI has risen to 70 in the third quarter of 2024.
Autonomous vehicles have witnessed one of the most significant technological advancements, including hardware and software systems. Self-driving cars must navigate unpredictable environments, necessitating agentic AI models that provide real-time adaptation through reinforcement learning, proactive decision-making, and the ability to learn from rare scenarios without relying on pre-programmed rules. Traditional AI systems are limited by their rule-based frameworks, which may falter in novel situations.
In the healthcare sector, agentic AI models are important in delivering personalized treatment plans that adapt to patients’ evolving conditions. These models can continuously learn and adjust to dynamic situations alongside predictive analysis capabilities, making them more effective than traditional AI models, which struggle to incorporate real-time patient feedback for improved accuracy.
In summary, agentic AI systems are transitioning from an industry buzzword to a tangible reality in the evolving application landscape. It will be interesting to see how these systems address the challenges that hinder adoption, including undocumented workflows, insufficient clean data, and the lack of integrated tooling.
How AI 2025 data center trends impact edge computing
Article Topics
agentic AI | AI | AI/ML | edge AI | edge computing | generative AI
Comments