WEF says ethical AI assists with predictive maintenance in Brazil’s public sector
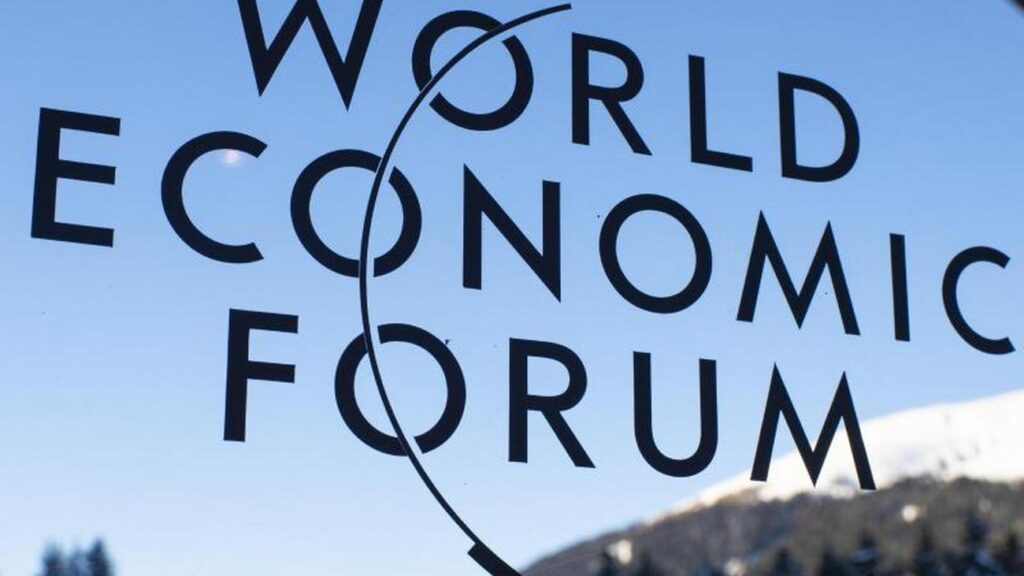
A pilot program organized by the World Economic Forum (WEF) in Brazil involving the country’s largest health complex and the subway network of São Paulo gave a series of recommendations for the ethical use and procurement of artificial intelligence (AI) in the public sector.
The Centre for the Fourth Industrial Revolution Brazil, a branch of the WEF, implemented principle-based guidelines for AI procurement in a program named ‘AI Procurement in a Box’. The framework seeks to set ethical considerations for AI-powered solutions and set a clearer adoption of AI by governments in the public sector. Using Brazil’s Metrô de São Paulo and the Hospital das Clínicas of the University of São Paulo for two separate pilot programs, the Center sought to uncover unique insights from each pilot program. For the Metrô de São Paulo, it looked at the challenges of implementing an innovation procurement procedure for the first time, and the unprecedented use of an algorithm impact assessment tool to identify and mitigate possible risks in the deployment of a predictive maintenance AI tool.
Using AI for predictive maintenance in subway systems
The Metrô’s objective was to purchase an AI-powered predictive maintenance system for online, real-time monitoring of rail tracks throughout the subway network. It would involve cameras on trains and sensors to enable deep learning and automated image processing to support decisions from Metrô’s engineering team to minimize delays caused primarily by maintenance, which totaled 1,840 minutes in the last four years affecting approximately 460,000 passengers.
The solution involved the adoption of a pre-commercial procurement procedure to allow the development of a new service, product, or innovative process when technological risk is involved. The Center came up with an algorithmic impact assessment model to fit Brazilian laws and regulations modeled after Canada’s Algorithmic Impact Assessment, which incorporated the main risks with the use of AI into the Metrô’s request for proposal.
From there, the pilot program would test for the following AI Procurement in a Box guidelines: selecting a needs-driven procurement process, incorporating relevant legislation and codes of practice, having a multidisciplinary team, and creating market engagement.
Upon completion, the accomplishments of the Metrô pilot program were the adoption of a mission-oriented pre-commercial procurement procedure to prototype and deploy a predictive maintenance algorithm; the first use of algorithmic impact assessments in Brazil, as recommended by Brazil’s National AI Strategy; and the creation of an independent board of specialists to assess risks associated with the project and lower asymmetries of information between the contracting entities.
Report conclusions and recommendations
The overall conclusions of the two studies ended with three suggestions, particularly for countries in the Global South that are lagging behind on AI governance frameworks, institutional and technical maturity, and skills for developing and deploying these technologies.
The Center says governments should self-assess their access to information technology (IT) infrastructure, appropriate data, and their institutional maturity and internal skill sets prior to acquiring AI. Secondly, it advocates for a mix of policy instruments like algorithmic impact evaluations and certifications from governments to ensure the responsible use of AI within their organization and expand these policies to internally developed AI models and systems that do not go through traditional procurement processes. Thirdly, the Center says governments should carefully consider the interactions between humans and AI models to mitigate both algorithmic bias and human-based bias.
Similar guidelines for ethical use of AI come from the public sector like the European Commission, the U.S. Department of Defense, and the private sector from Microsoft to IBM.
Article Topics
AI/ML | computer vision | edge AI | ethical AI | health | Industry 4.0 | predictive analytics | predictive maintenance | transportation | WEF
Comments