Webinar: Turning Large Dataset IP into AI “Gold”
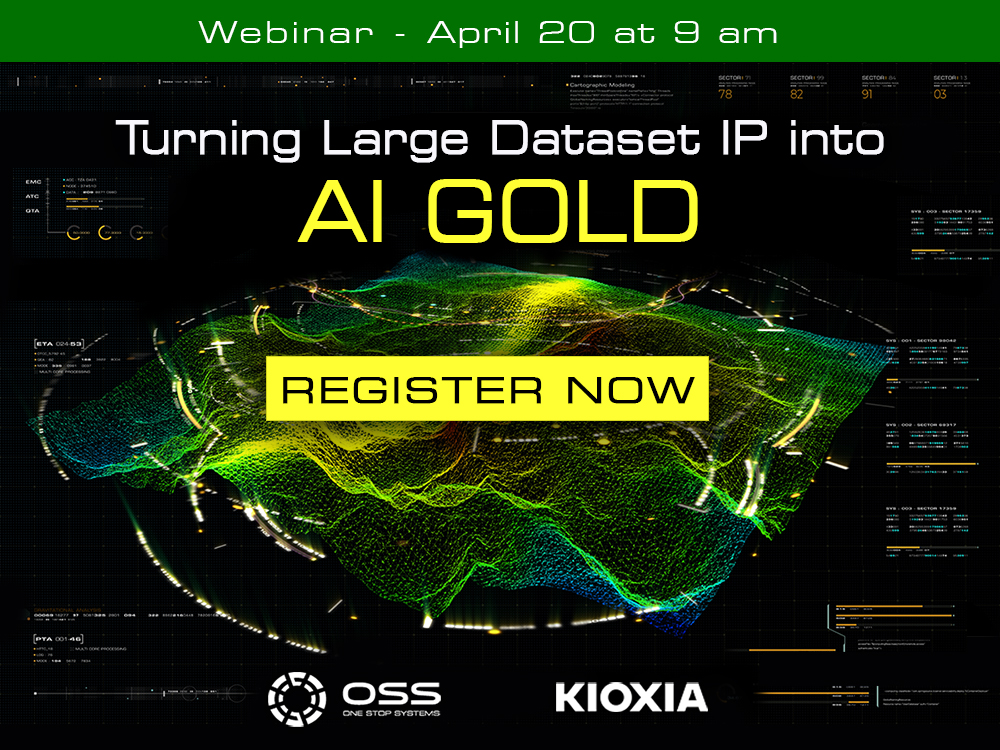
Collecting, encrypting, and creating value quickly from large AI datasets in Defense, Aerospace, Autonomous Vehicles and Security with no-compromise, AI Transportable infrastructure at the edge.
Speakers:
- Jim Ison, Chief Sales and Marketing Officer, OSS
- Matt Hallberg, Senior Product Marketing Manager of Enterprise NVMe, KIOXIA
All AI data workflows begin with acquiring and storing data before training AI models and deploying AI inference solutions. In edge applications, such as autonomous driving, military surveillance and video analytics, the proliferation of data from potentially thousands of high-bandwidth sensors and video sources requires high-capacity, high-endurance storage solutions in PCIe 4.0-based systems that can survive in harsh environments. These storage solutions also require high speed low latency data transfer performance to feed the AI compute engines.
In this webinar, speakers from OSS and KIOXIA will explain the state-of-the art in all flash AI data acquisition and storage systems that allow AI firms to collect, store and serve AI datasets on the edge where the data is generated and turn AI data quickly into an actionable, revenue generating asset with a particular focus on data security. They will describe how advancements in features, design, power and cooling have been made to allow these solutions to be deployed in rugged edge environments. KIOXIA will describe their advancements in NVMe technology and how they have created drives ideal for AI applications from the datacenter to the edge. Both companies will discuss a multi-tiered approach to data security, meeting even the most stringent FIPS 140-2 level requirements, at the drive, system or private network level to keep AI dataset IP secure.
Register Now!
American Tower opens newest edge data center in Pittsburgh
Article Topics
AI | AI webinar | edge computing webinar | edge event | edge webinar | KIOXIA | One Stop Systems | Turning Large Dataset IP into AI “Gold” | virtual event | webinar
Comments