The role of edge computing in expanding cloud AI deployments
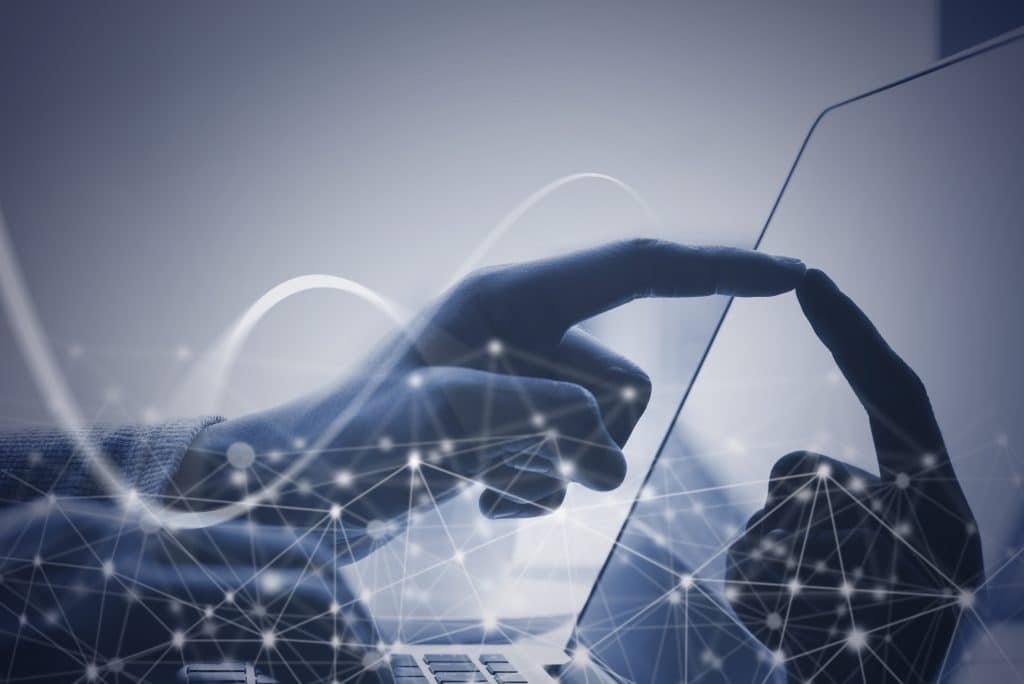
As the adoption of commercial AI solutions such as ChatGPT and Google Bard continue to shape the future of global business, many organizations are expected to increase spending on technologies intended to improve, optimize and scale AI-informed software deployments.
Data published in 2023 suggests that AI is now the biggest spend for almost 50 percent of leading tech executives across the economy, with experts believing individual leaders may increase spending by as much as 25 percent in the next 12 months. As AI tools continue to become more widespread across business sectors, teams must commit to enhancing core AI capabilities.
Currently, many technology providers may be limited to training AI models in centralized data centers, introducing undesirable latency to the delivery of AI-informed responses. However, the introduction of edge computing solutions to the landscape of AI could help to action this problem and support organizations in successfully expanding modern cloud AI deployments.
Reducing data transmission costs
For AI-informed systems to be effectively utilized on a commercial scale, stakeholders must ensure that solutions are optimized to perform calculations accurately and efficiently. While deployments designed to process information in centralized data centers can be effective to some extent, the latency introduced by such solutions can create issues as systems scale.
Of particular concern for large-scale operations is the sheer cost of continuous data transfers between remote data centers and user-facing applications. For organizations to consistently and efficiently perform remote calculations and transfer responses back to the user, leaders must factor significant network costs into the ongoing viability of newly deployed AI solutions.
By instead opting to develop edge AI systems, whereby calculations are performed close to the source of relevant data, businesses can significantly reduce expenses related to ongoing data transmission costs. Edge computing in this sense shows promise in helping developers expand novel cloud AI deployments with less need to worry about rising data transfer costs.
Enhancing data security practices
While the potential cost of proposed cloud AI expansions will no doubt factor into decisions regarding a project’s viability, the potential benefits of exploring edge AI solutions stretch far beyond financial incentives. Research suggests 65 percent of organizations are concerned about data privacy in new AI developments, with sensitive data potentially exposed to interference.
Cloud AI deployments reliant on data centers to collect and process data may be vulnerable to sophisticated cyber attacks, with global cyber attacks increasing by almost 40 percent in recent years. The more reliant systems are on transferring identifiable information between sources and data centers, the higher the potential risk of interception causing concern for end-users.
By ensuring all data is collected and processed locally by edge AI solutions, users face far less of a risk of their personal information being compromised. Sensitive data can remain secured within the confines of edge devices and receive further protection from considered physical security solutions. This can greatly reduce the attack surface exposed to potential hackers, enabling leaders in highly-secure environments to utilize AI-informed tools safely.
Minimizing operational latency
One of the primary benefits of cloud AI deployments for modern businesses is their ability to assist leaders in making data-informed decisions promptly and efficiently. This concept has become so widespread among C-suite executives that as many as 92 percent believe companies should leverage AI to support business decisions, with 79 percent already utilizing AI solutions.
However, if cloud AI solutions are to reasonably offer leaders a competitive advantage, tools must be optimized to deliver responses as promptly as possible. Edge AI developments can help leaders to achieve this goal by ensuring all processing is performed at the data source, enabling systems to deliver prompt insights with minimal latency in fast-paced environments.
Providing unparalleled flexibility
Finally, the decision to perform AI data analytics processes locally on edge hardware assists leaders in customizing solutions to suit the unique requirements of different organizations. AI models may be adjusted in keeping with the operational capabilities of specific edge devices, enabling in-house teams to continually optimize tools for integration with unique applications.
With calculations performed in a local environment, leaders can make informed decisions at a local level, removing a team’s reliance on remote cloud-based resources and enabling staff to make adjustments in response to changing demands. This additional flexibility can help to improve device reliability and aid leaders in expanding AI deployments of their own design.
Summary
As modern developments in the field of artificial intelligence continue to reshape businesses across most sectors of the economy, leaders must consider ways to optimize AI capabilities in line with expanding deployments. While models designed to communicate with centralized data centers can be effective in some cases, edge AI solutions bring numerous key benefits.
By developing systems in which calculations are performed locally on edge devices, leaders can reduce data transmission costs, enhance data security processes, minimize operational latency and enable teams to freely develop customized solutions. The adaptability that edge computing can add to modern AI tools may prove pivotal in expanding cloud AI deployments.
About the author
Sean Toohey is a freelance journalist and digital media specialist with extensive experience covering news, developments and emerging trends in AI and cloud-based technologies. His work for industry-leading organizations like Motorola focuses on the adoption and impact of smart technologies like AI, the Internet of Things and cloud computing on modern industries.
What is edge orchestration?
AI driving demand for inference computing needs
Article Topics
AI | edge AI | edge computing
Comments