Schneider Electric’s whitepaper unveils the future of data center design at the edge
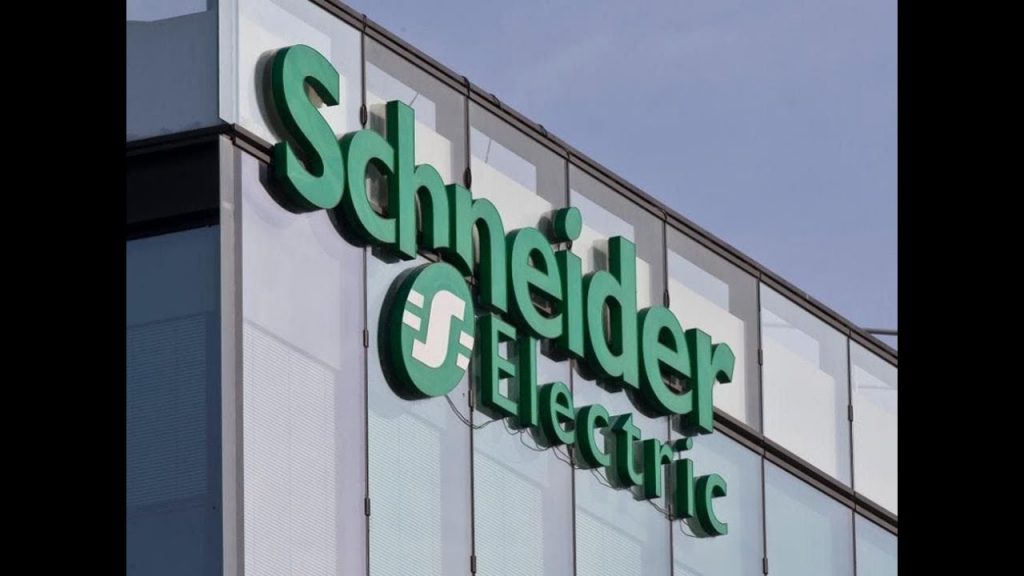
Schneider Electric recently launched a guide to addressing new physical infrastructure design challenges for data centers to support the shift in artificial intelligence (AI)-driven workloads, setting the gold standard for AI-optimized data center design.
Titled “The AI Disruption: Challenges and Guidance for Data Center Design,” the document provides insights into leveraging AI to its fullest potential within the data centers, including a forward-looking view of emerging technologies to support high density AI clusters in the future.
Steven Carlini, vice president, innovation and data center at Schneider Electric tells EdgeIR that AI includes a new ecosystem for data centers, as well as new server architectures, which does include core training models, but also deployment of inference models at the edge.
“If you look at IP equipment, traditional IP servers – one or two sockets – usually x86 processors, these servers for training, use a couple of CPUs, but that’s mainly to control the GPUs. Each server can have up to eight GPU accelerators. All of the GPUs within a cluster operate together, and you could have 10s of 1000s of GPUs, each one will have its own network and they function as a single device that are all networking together,” he adds.
“The aim is to put as many GPU accelerators into a chassis and as many chassis as possible into an IT rack, and as many racks as possible into a cluster. But you run into physical limits such as weight issues and space issues as these servers are heavier and deeper than traditional servers.
“If they have water cooling, then you have to deal with all the piping going in and out of the servers, and each server chassis will have up to six power supplies. This means there’s six power chords coming out of each chassis, which creates a huge challenge to put these into IT racks and put these IT racks together into a cluster.”
The whitepaper addresses how to solve these challenges, looking at the four key AI attributes and trends that underpin physical infrastructure challenges in power, cooling, racks and software management
Future of the data center in relation to the edge
As the demand for low-latency, real-time applications (e.g., IoT, autonomous vehicles, augmented reality) grows, there’s an emergence of edge data centers. These are smaller, decentralized data centers located closer to the edge of the network to serve local processing needs. Edge data centers can be found in various locations, including cell towers, industrial sites, or even within vehicles.
“For edge, you have the general purpose edge that you’re starting to see, you’re seeing extensions of the cloud, and telecom data centers that are multipurpose. We’re starting to see deployments at the edge becoming more multipurpose. You can deploy an IT rack in the carpark or basement or closer to the load, but it’ll serve multiple functions,” adds Carlini.
“If you’re running, for example, some kind of autonomous driving, and you’re taking all of the data from the automobiles into the edge data center and have to make real time decisions, that data center cannot be very far from the actual data and the vehicles.”
Both centralized and edge data centers will have their roles to play, with a focus on optimizing performance, reducing latency, and meeting the evolving demands of emerging technologies and applications.
Digital twins
In the evolving landscape of technology, the concept of digital twins has emerged as a transformative force, reshaping industries from manufacturing to healthcare. A digital twin is a virtual replica of a physical object, system, or process, providing a real-time, data-driven representation that allows for analysis, simulation, and optimization.
When asked about digital twins, Carlini says: “It’s much better practice to design it digitally before you implement it in the world physically, and the ability to run a digital simulation of your environment and to actually make changes, you’ll know what the effect will be.
“It takes a lot of the risk out of designing it physically because you have a better idea of what’s going to happen. We’re seeing much more digital twin designs because of the densities that we’re dealing with in these racks and clusters.”
Carlini explains that with digital twins, businesses can dial in the kind of data center for efficient and sustainable operations before deploying it physically.
“The white paper does a good job of explaining the differences between training models and inference models. With inference models, you’re taking that model that you’ve trained, and you’re deploying it in the field to make the decision. The inference model is the work that’s being done in action,” he concludes.
EDGX, BrainChip unite to create data processing units for space
Article Topics
AI | data center | digital twins | edge | Schneider Electric
Comments