Navigating the cloud while optimizing AI architecture for maximum efficiency
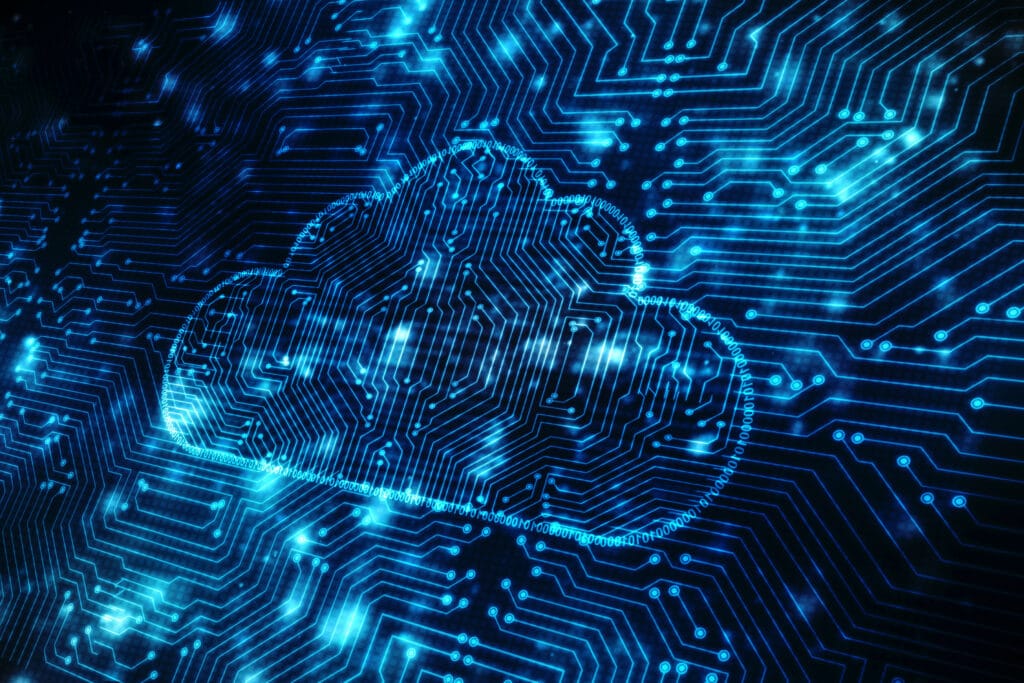
By Mark Lewis, head of product marketing at Zadara
An organization’s choice of hybrid-cloud platform to build and sustain its Artificial Intelligence (AI) initiatives has emerged as a key consideration for those businesses looking for the best ways to harness the full potential of their data. And while it may seem like AI is everywhere and all businesses are utilizing it, that is not the case. While AI developments started as early as the 1950s, widespread adoption is still in its nascent phase with many organizations trying to get their arms around how to best utilize it for business benefit.
AI, even after an organization understands how and where it will use it, requires a flexible infrastructure to host it. It’s at this point that the question becomes – what is the best approach for integrating cloud computing into AI initiatives? Is there a one-size-fits-all cloud architecture that can seamlessly accommodate all phases of AI lifecycle development and deployment?
While cloud computing offers a wealth of resources and scalability, there is no singular blueprint that suits every AI project. Instead, the optimal approach depends on various factors including the nature of the AI application, data requirements, computational complexity, and of course, budget constraints.
One fundamental consideration is the choice between public, private, or hybrid cloud. Public clouds, such as those offered by the hyperscalers – Amazon Web Services (AWS), Microsoft Azure, and Google Cloud – provide extensive scalability making them ideal for big AI projects with fluctuating and ongoing resource needs.
Private clouds, on the other hand, offer enhanced security, customization, and cost effectiveness, making them well-suited for organizations with strict compliance requirements or sensitive data. Hybrid cloud solutions combine the benefits of both public and private clouds, allowing organizations to leverage the flexibility of the public cloud while maintaining control over critical data and applications.
Cloud technology is built on a scalable infrastructure that assists organizations in escalating and decreasing their compute and storage resources to meet the demands of their AI workloads. Whether organizations are data processing or training AI models, it is important to onboard the right infrastructure that can adjust in real time to handle those fluctuating resource requirements without compromising performance, reliability, security or the budget.
Considerations
AI workloads vary widely in terms of computational complexity, data size, and performance requirements. Customizable solutions allow organizations to tailor their cloud infrastructure to suit their specific AI use cases. Whether organizations need high-performance GPUs for deep learning tasks, storage for real-time data processing, or low latency networking for distributed AI workloads, a range of configuration options to accommodate diverse AI requirements is a fundamental consideration.
Effective data management and security are paramount for successful AI initiatives. Data management features that include encryption, data replication, and data protection, to ensure the integrity, availability, and confidentiality of AI datasets are non-negotiables.
Many organizations operate in hybrid or multi-cloud environments, where AI workloads may span across on-premises infrastructure, public clouds, and private clouds. To support these complex deployment scenarios, hybrid and multi-cloud solutions that provide seamless integration with the hyperscalers – AWS, Microsoft Azure, and Google Cloud – enable organizations to take advantage of the scalability and agility of public clouds while maintaining control over their most critical data.
Finally, cost is a significant consideration for organizations deploying AI workloads in the cloud. A transparent pricing model can provide predictability, and cost-efficiency for AI deployments. Flexible pricing options, including pay-as-you-go and subscription-based models, allow organizations to align their cloud expenditures with their actual usage patterns and budgetary constraints, optimizing cost-effectiveness and ROI for AI initiatives.
Once an AI model is trained, an organization’s focus typically shifts to deployment, where the choice of cloud architecture often depends on factors such as latency requirements, geographical distribution, and once again, cost. For latency-sensitive applications, deploying AI models closer to end-users using edge clouds can minimize latency and improve user experience. Cloud providers that offer edge AI solutions enable organizations to deploy AI models directly onto edge devices for real-time inference or AI that analyzes incoming data and generates predictions or responses instantaneously.
Final thought
While there is no one-size-fits-all cloud architecture for AI, organizations have choices in how they leverage cloud computing to accelerate their AI development, and deployments. By assessing their requirements and as well as the deployment options offered by cloud providers, organizations can design a scalable, efficient, and cost-effective AI architecture that unlocks the full potential of their data.
About the author
Mark Lewis is responsible for product marketing at Zadara, based near to London, UK. He has over three decades of experience across engineering, sales and marketing having previously held international leadership roles at large corporate and fast-growth organizations including BT, Sun Microsystems, Riverbed, Nutanix and VMware.
Comments