Living on the edge: the new horizon for analytics
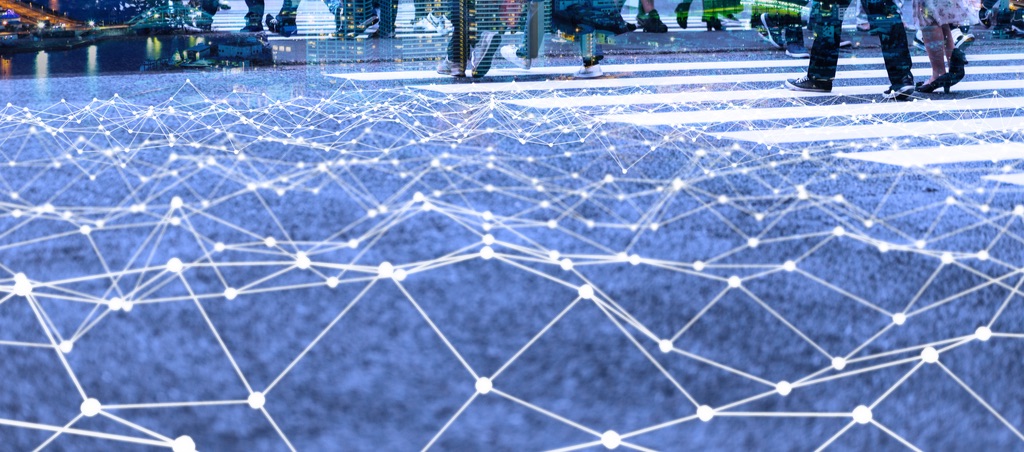
This is a guest post by Ravi Shankar, senior vice president and CMO at Denodo.
As technology evolves, so do new problems that require a new approach. With the advent of smart devices such as intelligent switches, thermostats and third-generation voice assistants, data volumes have exploded and reduced the efficacy of centralized computation and analysis. Edge computing solves this problem by making these smart devices even smarter by helping them to process their data to suffice their needs closer to them at the edge node, and then transmit only the data that is required for centralized computation. This approach not only improves the efficiency of the edge devices but also that of the centralized analytical systems. Given the promise of edge computing, it is poised to take off as one of the most important technology trends in 2020 and beyond.
For example, Google’s Nest uses machine learning algorithms to learn when the residents are home or away on weekdays or weekends, based on daily temperature adjustments, over the course of time. With this information, Nest can adjust the temperature by itself throughout the week and weekends. Nest’s mix of edge and centralized processing highlights an interesting challenge for enterprise data management.
Traditionally, enterprises have adopted an architecture of analyzing data and deriving intelligence from it using a centralized approach. For instance, data warehouses, the workhorse of business intelligence, are well-known central repositories that can turn raw data into insights. The process, known as ETL, extracts data from operational systems, transforms it into the appropriate format, and then loads it into the data warehouse.
For many years, this architecture has proven effective. But in the era of edge devices, traditional physical data warehouses are losing their luster as the central source of the truth. This is because they can only store structured data as the world moves today to a flood of unstructured data. Also, the volume of data is growing exponentially. It has become so vast that for many use cases, it is no longer economically feasible to store all of the data in a single data warehouse. To overcome these challenges, companies transitioned their central repository to cheaper alternatives like Hadoop, which can also store unstructured data.
Despite these evolutions, it is still undesirable from a performance and cost perspective to collect all of the information generated across multiple devices residing in various locations across the world into one central repository that is thousands of miles away. Nor can the information be efficiently analyzed for intelligence by this central system, and then used to make recommendations all the way back to the devices for optimal performance.
So, what’s missing?
In our view, it is the technology to execute the compute function closer to the devices itself. The emergence of edge computing architectures enables devices to send the data they generate to an edge node, or a system that is closer to the devices, for analysis or computational purposes. In this way, the devices can gain the intelligence needed much faster from the edge node than they otherwise would when connected to a central system.
In this setup, the edge nodes are connected to the central system, so they transmit only the information that is needed for the central system to analyze across all of the various devices. As a result, there is a duality of computation in which some computation happens at the edge nodes to the extent it is needed for local operation and, at the same time, data is transmitted to a central analytical system to perform the holistic analysis across all of the enterprise systems.
The ability to smartly filter only the needed data at the edges and transport just the reduced data to a centralized system is fortunately available today. By reducing the data moved as much as 80%, data virtualization can perform this selective data processing and delivery in real-time without having to replicate the data within it.
As data comes in from various devices, the data virtualization instance that sits at the edge node closer to these devices, integrates the disparate data from them and then extracts just the results. It then delivers them to another instance of data virtualization that sits in the central location, closer to the data consumers, who use reporting tools to analyze the results. So, a network of data virtualization instances, some at the edge nodes, connected to a central data virtualization instance, in a multi-location architecture, complete the edge computing framework.
Why it’s smarter to be on the edge
The biggest benefit of edge computing is time savings. Over the past several years, two aspects of the technology have evolved much faster than the others—storage and compute. Today’s mobile phones have more memory and compute power than desktop PCs from 30 years ago had. Yet, one aspect of the technology that has not evolved as fast as the bandwidth to transmit data, as it still takes minutes and hours for data to move from one location to another. With the devices moving farther and farther away to the cloud and across continents, it becomes imperative to transmit the minimal amount of data as possible to improve the overall efficiency.
By delegating compute to the edge, these devices will learn and adjust in real-time rather than being slowed down by the transfer of information to and from a central system . Data virtualization reduces the bandwidth requirements as well as storage costs today by as much as 80%.
About the author
Ravi Shankar is senior vice president and Chief Marketing Officer at Denodo, a leading provider of data virtualization software. For more information visit the Denodo website or reach out to us on Twitter.
DISCLAIMER: Guest posts are submitted content. The views expressed in this blog are that of the author, and don’t necessarily reflect the views of Edge Industry Review (EdgeIR.com).
Article Topics
big data | data virtualization | data warehouse | Denodo | edge AI | edge analytics | edge computing | IoT
Comments