Infrastructure takeaways from NVIDIA GTC 2025
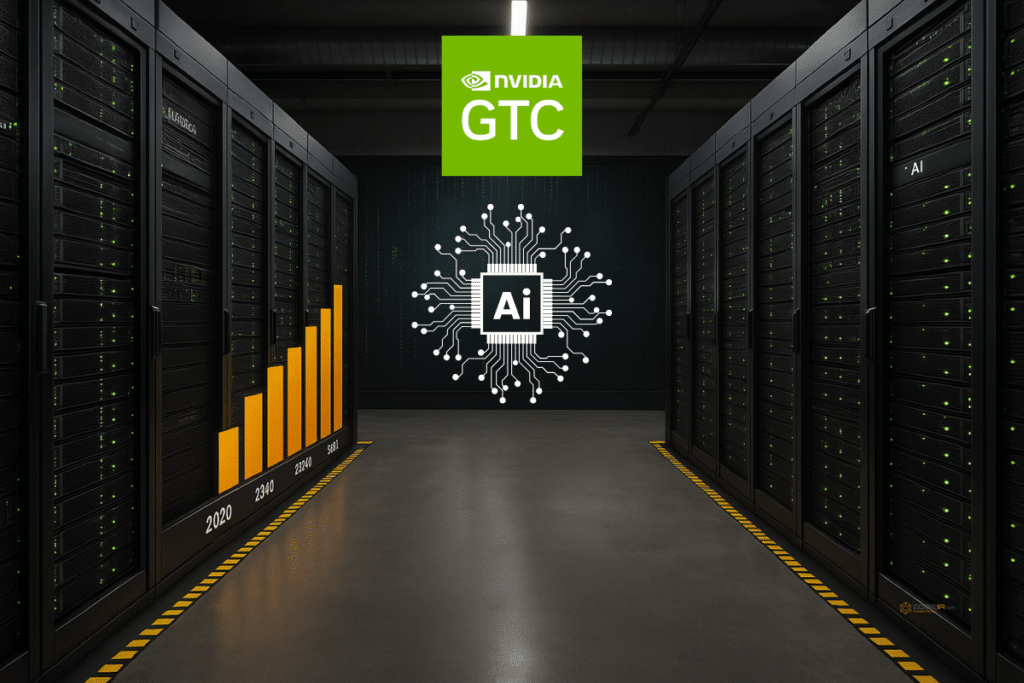
Summary: The recent NVIDIA GTC conference included some interesting data points and insights on data centers that provide insights into how digital infrastructure will need to be built to support the coming expansion in AI.
Details: There was a big shift in thematic focus from AI training to AI inference and agentic AI. Agentic AI will need more tokens (in the hundred times range compared to today’s ) to conduct the AI reasoning that will drive next-generation AI applications (along with the inferencing), with compute needing to keep up at the same velocity. And that is the basis for the main infrastructure takeaway coming out of the NVIDIA event. NVIDIA CEO Jensen Huang put up a few charts that suggest up to a trillion dollars of data centers capacity (not sure how that is measured or if inclusive of chips, servers, racks, etc.) will be needed by the end of the decade to support what is believed to be in the pipeline. The big numbers continue to get thrown around, but at the core of it, NVIDIA’s belief is that general-purpose computing is running its course. Of course, that does not mean it is going to disappear and go away. But NVIDIA sees an inflection point coming as software applications transition from file retrieval to retrieving and generating tokens at high volumes. The implication being that so much more computing capacity and infrastructure is going to be needed to support this. And it is not just about capacity, but how data centers are built, hence the idea of AI factories, rather than data centers. The other aspect of the AI factory concept is the belief that it is not just about chips. NVIDIA is positioning around the stack, inclusive of software, hardware and networking. So these AI factories will be built, NVIDIA hopes, to house its entire technology stack.
Impact: The importance of data centers capacity cannot be understand and even Huang himself noted on stage that ‘we are a power limited industry … our revenue is associated with that’. So it is not just about capacity, but more energy efficiency, which is a focus of each new generation of chips.
GPU technology cycles: Speaking of GPU generations, NVIDIA rolled out the rather ambitious plan to update its technology frequently. The goal is to update the GPU product every year, with a new architecture every 2-3 years. Huang explicitly noted that land and energy will also be needed 2-3 years in advance (likely further), speaking to the corresponding infrastructure requirements that are going to drive this trillion dollars of data centers capacity. To give some sense for where NVIDIA expects things to be going … the next line of GPUs, called Rubin, are going to be designed for 600kW per rack and made available by 2027 (if it hits this target), but this will depend on the infrastructure and technology being available to support it.
Data point around hyperscale top four GPU consumption: A useful data point shared was GPU shipments to hyperscalers (the top four in the US). Hopper shipments to this group was 1.3m GPUS, at its peak, while Blackwell shipments have reached 3.6m in just the first year. Blackwell is now said to be in full production.
GPU generations and market implications: Another interesting comment around infrastructure was when Huang said rather boldly that Blackwell would render Hopper basically useless. He seemingly backtracked while quipping that he was the chief revenue destroyer, noting that Hopper will make sense in some use cases. That last comment is key and at the heart of how things will shape out. Will people always need the latest and greatest, and what can older generation GPUs be used for? Older generation GPUs should go a long way to absorbing some of the demand that is out there, while lengthening the shelf life and monetization window for plenty of expensive hardware.
Angle: There continues to be plenty of debate, chatter, worry and consternation about AI demand and what it means for the data centers and hyperscale infrastructure industries. Notably, Huang pointed out that AI started in the cloud and on hyperscale platforms because it needed copious amounts of infrastructure. Cloud made it available relatively quickly and efficiently. NVIDIA expects this will continue even as hyperscalers look to get into the GPU game and sell cheaper options and alternatives. But it is expected that the sector is going to need a lot more and it will be pushing the limits of what is feasible and available in a reasonable time frame. For those worried about demand fading off or the requirements not being as big as they are believed to be, those are more likely to be unfounded over the long-term. Densities will continue to rise and more chips are going to be deployed on hyperscalers platforms and in data centers. The technology envelope will continue to be pushed. The question looks now to be more about timing and the feasibility of supporting such huge capabilities. If looking at NVIDIA’s sharp timelines, it does not look like there is going to be enough capacity. There will need to be time for the various alternative energy sources to be evaluated, researched and deployed. Purpose-built facilities still take time to stand up and that can and will only move forward if the energy is there. More likely, things are going to play out over a longer period of time than NVIDIA might believe. Delays and bumps in the road are likely to still arise. It will not necessarily be a bad thing as infrastructure needs a lot more time to be ready. It certainly will not move as fast as NVIDIA thinks the technology will.
Analysis:
Outside the NVIDIA universe of large and power-hungry chips that cost anywhere from $30,000 to an estimated $70,000 for next-generation designs, there is a world where power supply and cost constraints require a different approach to system design. Still, as in the data center-focused world that the NVIDIA Hopper and Blackwell chips will reside in, edge AI is undergoing rapid evolution. More efficient LLM models are certainly one aspect of this, but AI at the edge encompasses many more forms of AI (and models) than just generative AI. One example of this is Qualcomm’s acquisition of Edge Impulse, which marks an important phase in the development of edge AI. When a company like Qualcomm, with its presence in smartphones and IoT markets, makes a significant investment in edge AI, it will have a knock-on effect of startup valuations and venture investment. Financial results from ARM, another leading chip design firm for IoT and edge devices, suggest that even at the other end of the chip cost spectrum, companies can also profit from the edge AI era.
Jim Davis contributed to this article.
About the author:
Phil Shih is Managing Director and Founder of Structure Research, an independent research firm focused on the cloud, edge, AI and data center infrastructure service provider markets on a global basis.
Article Topics
agentic AI | AI factories | AI inference | AI/ML | data centers | edge AI | edge computing | GPU | hyperscale infrastructure | Nvidia | NVIDIA GTC 2025
Comments