How does edge computing enhance the performance and efficiency of AI solutions?
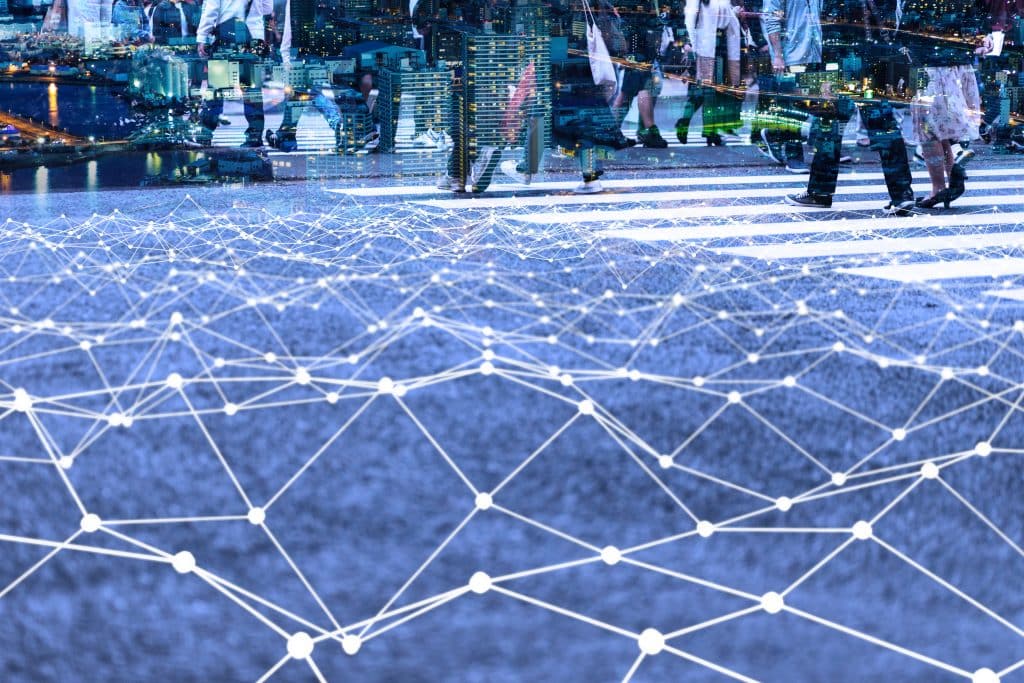
By Ben Hartwig, a Web Operations executive at InfoTracer
Artificial intelligence (AI) has emerged as a transformative technology, revolutionizing various industries and applications. From autonomous vehicles to virtual assistants, AI-powered solutions are becoming increasingly prevalent. However, the widespread adoption of AI also poses challenges, particularly in terms of performance, latency and data privacy.
To overcome these hurdles, some organizations are turning to edge computing. Edge computing brings computation closer to the data source and can enhance the performance and security of AI solutions.
The role of edge computing in optimizing AI solution performance
Traditional AI models often rely on centralized cloud infrastructure, which necessitates sending data back and forth between the device and the cloud server. This back-and-forth communication introduces latency and can be particularly problematic in real-time applications.
By leveraging edge computing, AI models can be deployed directly on edge devices, reducing the need for continuous data transmission to the cloud. This enables faster processing and decision-making, leading to improved performance in time-sensitive applications such as autonomous vehicles, industrial automation and robotics.
Harnessing edge computing for enhanced privacy and security in AI solutions
Privacy and security are critical concerns in the era of AI. Edge computing plays a crucial role in addressing these concerns by reducing reliance on cloud services for data processing. With edge computing, data is processed locally on the device or at the network edge, minimizing the need to transmit sensitive information to external servers.
This approach significantly enhances privacy by limiting the exposure of personal and sensitive data. Additionally, IP address lookup can be performed at the edge, allowing AI solutions to verify and authenticate users without relying on central servers. This further strengthens security by reducing the attack surface and potential vulnerabilities associated with centralized cloud infrastructure.
Reducing latency and enhancing real-time responsiveness with edge computing
Latency, or the delay between data transmission and processing, is a critical factor in AI applications. In scenarios where real-time responsiveness is crucial, such as autonomous vehicles or industrial control systems, any delay can have severe consequences. By deploying AI models on edge devices, edge computing minimizes latency by processing data locally.
This reduces the round-trip time to the cloud and enables faster decision-making. For example, an autonomous vehicle can quickly analyze sensor data and respond to immediate situations without waiting for instructions from a distant cloud server. The reduced latency achieved through edge computing enhances the overall performance and reliability of AI solutions.
Edge computing as a catalyst for decentralized and distributed AI systems
Edge computing also plays a pivotal role in enabling decentralized and distributed AI systems. Traditional AI architectures typically rely on a centralized cloud infrastructure, which can become a single point of failure and limit scalability. In contrast, edge computing allows AI models to be distributed across multiple edge devices, forming a decentralized network.
This distribution enables fault tolerance, as each device can continue processing data even if other devices fail. Furthermore, edge devices can collaborate and share information locally, enabling collaborative AI models and reducing the need for constant communication with a central server. These decentralized and distributed AI systems foster scalability, flexibility and adaptability.
Overcoming network limitations in AI solution deployment
Deploying AI solutions in environments with limited or unreliable network connectivity can be challenging. Edge computing offers a solution by moving computation closer to the data source. In scenarios such as remote industrial sites or rural areas, where connectivity is sparse, edge devices can perform AI processing locally without relying on continuous network access.
This reduces the dependence on stable network connections and allows AI solutions to operate seamlessly, even in challenging network conditions. By overcoming network limitations, edge computing enables the deployment of AI solutions in previously inaccessible environments, unlocking new possibilities for various industries.
Final thoughts
Edge computing is revolutionizing the field of artificial intelligence by enhancing the performance and efficiency of AI solutions. It optimizes performance by reducing latency and enabling real-time responsiveness. Moreover, edge computing addresses privacy and security concerns by minimizing data transmission to centralized servers and enabling IP address lookup for authentication.
Additionally, edge computing overcomes network limitations, enabling AI solution deployment in remote and challenging environments. As AI continues to evolve, edge computing will play a crucial role in unlocking its full potential while ensuring privacy, efficiency and real-time capabilities.
About the author
Ben Hartwig, a Web Operations executive at InfoTracer, takes a comprehensive approach by considering the entire system. He authors security guides that address both the physical and cyber aspects.
DISCLAIMER: Guest posts are submitted content. The views expressed in this post are that of the author, and don’t necessarily reflect the views of Edge Industry Review (EdgeIR.com).
Article Topics
AI/ML | data sovereignty | edge AI | InfoTracer | privacy | security
Comments