Edge AI vs. Cloud AI: Understanding the benefits and trade-offs of inferencing locations
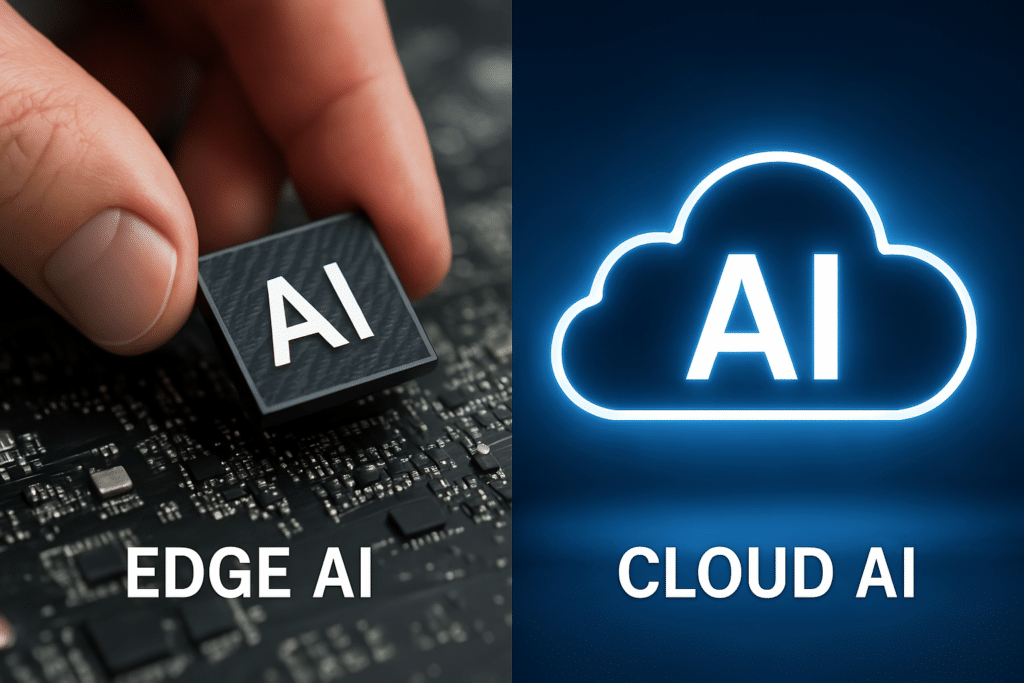
Ellie Gabel, Associate Editor for Revolutionized.com
As artificial intelligence gains momentum and people explore various use cases, inference locations are among their chief concerns. These are where AI decisions, predictions or other outputs occur. The main thing to determine is whether the edge or the cloud makes a more suitable inference location.
Latency requirements
As experts assess inferencing locations, they should consider whether their planned applications require real-time or similarly speedy responses. That will inform some of their decisions about latency, which is when AI receives input and responds by providing output.
Choosing the edge as an inferencing location is an excellent way to reduce latency because processing happens directly on AI-enabled devices. The alternative is sending the input to the cloud for processing, which takes longer. When people need the fastest functionality possible, edge AI is an ideal solution, but cloud-based processing may suit them if they do not mind slightly longer latency.
The edge is also appropriate for areas with limited connectivity. Industry leaders in sectors including mining and oil and gas have discovered AI can streamline workflows and solve problems. However, connectivity problems can restrict seamless transmissions to the cloud. They can bypass that issue if processing occurs directly on the device.
Budget and bandwidth
Transferring large amounts of data to the cloud for processing can get expensive. When budgets are a concern for those with decision-making capabilities, edge computing can be more cost-effective because processing occurs locally.
Conversely, cloud AI applications require data to go to and from the processing locations, requiring significant bandwidth. That approach is often more expensive in the long term, so leaders should calculate the expected costs into their overall budgets. One notable statistic was that they waste 32% of what they spend on their cloud services.
That does not necessarily mean they should consider cloud AI out of the question. However, it is wise for the involved parties to determine what they will periodically spend on specific services. Then, they should assess whether those possibilities represent money well spent.
A potential downside of edge devices is their limited processing capabilities. The cloud offers extensive processing power and storage. Additionally, if the company already relies on it for numerous other applications and leaders are familiar with the infrastructure, it could make more sense for them to focus on the cloud.
In one example, Deutsche Bank leaders migrated 260 applications to the cloud as part of plans to incorporate generative AI into various facets of its business. Decision-makers believed the provider’s presence as a well-established entity in cloud and AI technologies would give the bank the support to proceed with its plans. However, vendor lock-in is a potential downside that could interfere with flexibility.
Security concerns
Conversations about security also lead some to select edge or cloud AI inference locations. The cloud’s processing location is more distant than edge devices, but that also means cybersecurity issues might arise during transit or because of a provider’s negligence. At the same time, the cloud’s built-in security features can create safe inference locations if users understand their functionality.
A 2025 report estimates edge computing spending will hit $380 billion by 2028, and analysts mentioned AI as one of the driving factors. They split 1,000 potential enterprise use cases into six technological domains and found that artificial intelligence was the second fastest-growing after augmented reality. The researchers also mentioned that improved security makes edge AI opportunities attractive to many parties.
The local processing of AI data on edge devices gives professionals more control and oversight, letting them keep security tight. It can also make verifying that the information usage aligns with necessary regulations easier. Alternatively, edge devices collectively expand the potential attack surface, requiring the organizations using them to prioritize cybersecurity.
Examine Individual Circumstances
Since there is no universally acceptable answer to whether enterprises should use cloud AI or the edge, decision-makers should consider the aspects unique to their situations and goals. That personalized approach encourages them to scrutinize the specifics before acting.
About the author
Ellie Gabel is a freelance writer as well as an associate editor for Revolutionized.com. She’s passionate about covering the latest innovations in science and tech and how they’re impacting the world we live in.
NTT debuts breakthrough AI chip for real-time 4K inference at the edge
Article Topics
AI inference | AI infrastructure | cloud AI | cloud vs edge | edge AI | enterprise AI | latency optimization
Comments