Data-rich computing: M.2 meets AI at the edge
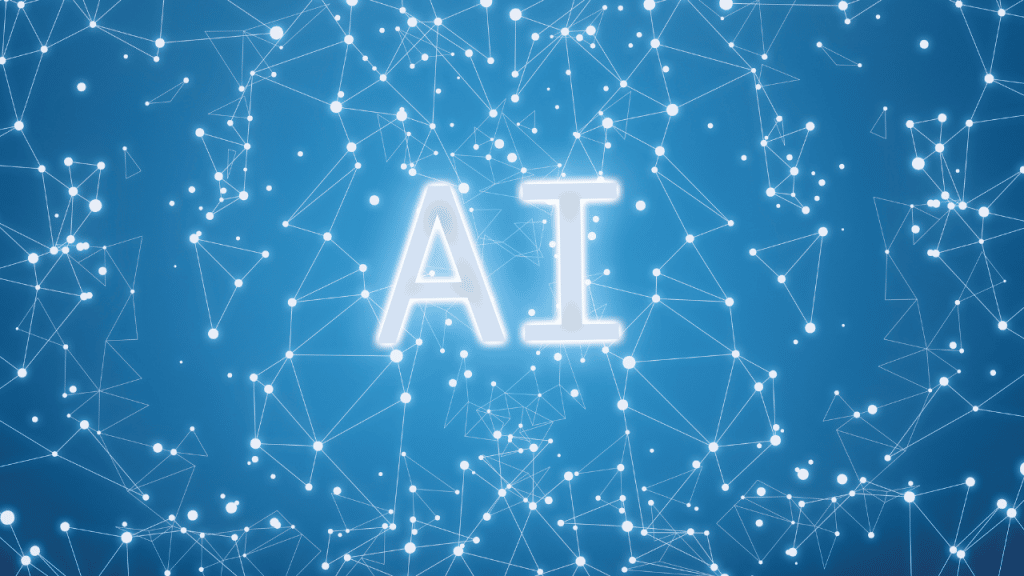
By Dustin Seetoo, director of product marketing, Premio
The proliferation of data — as well as the applications it drives — is creating seismic shifts. These shifts require specialization to create systems more capable of interacting with AI workloads, both efficiently and on-demand.
For system designers, this means that yesterday’s performance acceleration strategies may no longer fit the bill. While CPU/GPU-based designs have helped manage the slowing of Moore’s Law, these processor architectures are now finding it difficult to keep in step with the real-time data requirements inherent to automation and inference applications. This is particularly true in more rigorous non-data center scenarios. Combined with the mounting challenge to meet price-performance-power demands, it’s more critical than ever that performance acceleration consider compute, storage, and connectivity. All these factors are necessary to effectively consolidate workload close to the point of data generation, even in rugged settings where environmental challenges are detrimental to system performance.
This is where M.2 form-factor accelerators come into play for eliminating performance barriers in data-intensive applications. A powerful design option, M.2 accelerators offer system architects domain-specific value to match the exact requirements of AI workloads. In contrast to a comparable system using CPU/GPU technologies, an M.2-based system can manage inference models significantly faster and far more efficiently. These increases are driving innovative system design perfect for the rugged edge where more systems are deployed in challenging, non-traditional scenarios, and where purpose-built systems offer immense opportunity. Here there is a clear differentiation between a general-purpose embedded computer and one that’s designed to handle inferencing algorithms by tapping into more modern acceleration options like M.2 acceleration modules.
Diving into M.2 and domain-specific architectures
Accelerators deliver the considerable data processing required, filling the gaps caused by the deceleration of Moore’s Law which for decades was a driving force in the electronics industry. This long-established principle asserts that the number of transistors on a chip will double every 18 to 24 months. When it comes to AI, however, industry experts are quick to point to signs of wear in Moore’s Law. Silicon evolution in and of itself cannot support AI algorithms and the processing performance they require. To balance performance, cost, and energy demands, a new approach must feature domain-specific architectures (DSAs) that are far more specialized.
Customized to execute a meticulously defined workload, DSAs provide a fundamental tenet for ensuring performance that facilitates deep learning training and deep learning inference. With M.2 accelerators as an example, DSAs drive inference models 15 to 30 times faster (with 30 to 80 times better energy efficiency) than a network relying on CPU/GPU technology. While general-purpose GPUs can provide the massive processing power necessary for advanced AI algorithms, they are not suitable for edge deployments, particularly in remote or unstable environments. In addition to the upfront cost of the GPU itself, power consumption, size, and heat management drawbacks equate to even greater operating costs. M.2 acceleration modules and specialized accelerators (TPUs from Google, for example) are power efficient, compact, and purpose-built for running machine learning algorithms with incredible performance at the edge in a smaller form factor and with less power budget.
Why M.2?
Also known as the Next Generation Form Factor interface, M.2 was developed by Intel to provide flexibility and robust performance. M.2 supports several signal interfaces such as Serial ATA (SATA 3.0), PCI Express (PCIe 3.0 and 4.0), and USB 3.0. With a variety of bus interfaces, M.2 expansion slots are highly adaptable to different performance accelerators, storage protocols, I/O expansion modules, and wireless connectivity.
M.2 also offers both legacy and contemporary compatibility via support for SATA and NVMe (Non-Volatile Memory Express) storage protocols. Legacy standard SATA contains an Advanced Host Controller Interface (AHCI) and is defined by Intel as the storage protocol optimizing data manipulation via spinning metal disks in HDD (hard disk drive) storage. NVMe offers an alternative, designed to fully take advantage of NAND chip (flash) storage and the PCI Express Lane for extremely-fast solid-state drive (SSD) storage.
Performance accelerators have also embraced the M.2 form factor, benefitting from its powerful and compact interface. These include AI accelerators, memory accelerators, deep learning accelerators, inference accelerators, and more. Such specialized processors are dedicated to AI workloads, providing an improved power-to-performance ratio.
Unlocking AI with real-time data performance in more environments
Data is key to today’s business innovation, and more importantly, the ability to deliver cognitive machine intelligence. Whether powering advanced telematics or smart kiosks, on the factory floor, or driving passenger and surveillance services in infrastructure facilities like train stations and airports, data is all around us and is most valuable when it can be uncovered, captured, evaluated, and used in real-time.
Myriad industries are eager to make the most of data to create new services and enhance business decisions but in many rigorous industrial environments, processing small automated or AI tasks at the data center level is just too inefficient to provide true value. Power consumption and costs are too high in this legacy centralized compute structure due to excessive, albeit necessary, use of compute, bandwidth, and storage resources. Further, high latency mean performance takes a hit and insufficient data privacy makes for another headache.
Data growth, combined with edge computing environment complexities, is driving the AI computing framework away from general CPU/GPU options and toward specialized accelerators based on domain-specific architectures that use the M.2 standard — options that are smaller and more power-efficient. It’s a strategy to address a data challenge that is complex, real, and not going away. As the amount of IoT (Internet of Things) and IIoT (industrial IoT) devices increases, so does the volume and velocity of data they generate. Application designers and developers must recognize an urgent need for performance acceleration that resides closer to data sources and is purpose-built for the task at hand – particularly as edge computing hardware is deployed to cope with data processing and alleviate related burdens in data centers and in the cloud.
About the author
Dustin Seetoo is director of product marketing for Premio. Premio is a global solutions provider specializing in the design and manufacturing of computing technology from the edge to the cloud.
DISCLAIMER: Guest posts are submitted content. The views expressed in this post are that of the author, and don’t necessarily reflect the views of Edge Industry Review (EdgeIR.com).
Article Topics
edge AI | edge hardware | Google | GPU | IIoT | IoT | M.2 | Premio | TPU
Comments