Breaking the cost barrier: How enterprises can affordably scale AI at the edge
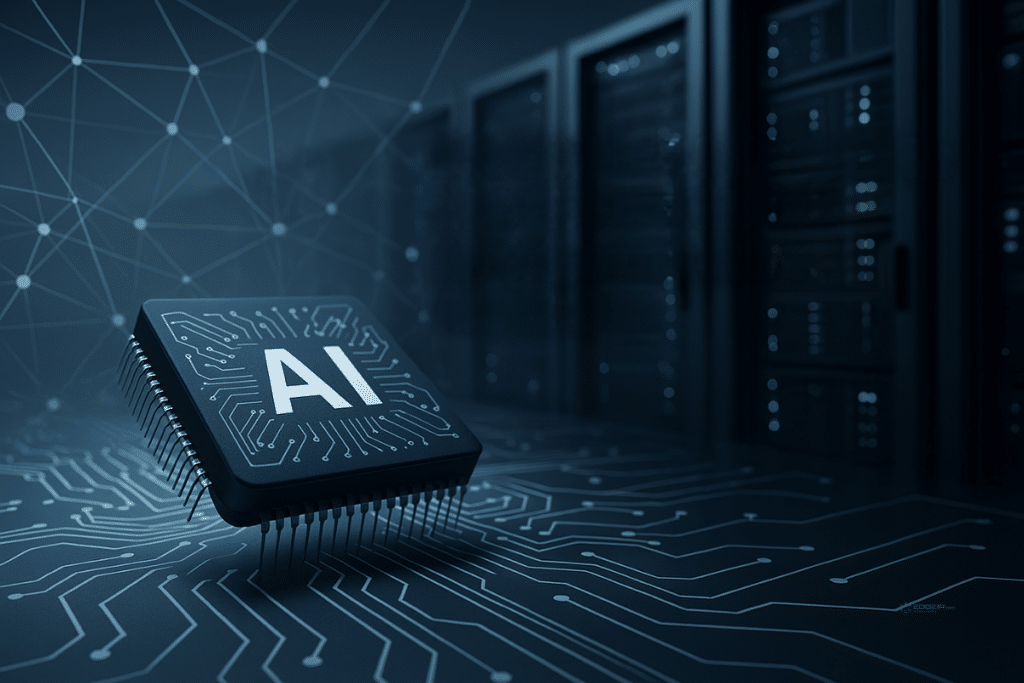
By Kevin Cochrane, Chief Marketing Officer, Vultr
2025 has been dubbed the “Year of Edge AI.” From smart manufacturing and autonomous vehicles to retail analytics and healthcare diagnostics, AI at the edge is transforming industries by bringing real-time intelligence closer to where data is generated. Despite its vast potential – faster decision-making, improved efficiency, and enhanced customer experiences – the high cost of deploying and scaling AI at the edge remains a significant challenge.
With the most advanced AI-driven organizations planning to put 200 models into production this year, edge leaders are grappling with managing expensive hardware, inefficient software stacks, and unpredictable infrastructure costs. Here is a practical playbook to overcome those challenges and unlock the full potential of edge AI.
The biggest cost drivers of edge AI
It’s important to understand where costs tend to pile up. Edge AI deployment comes with several hidden cost factors that businesses must navigate carefully, including:
- Specialized AI hardware: Many organizations overspend on high-end GPUs and CPUs without thoroughly assessing workload requirements. While top-tier processors deliver high performance, they may not always be necessary for every AI application.
- Infrastructure complexity: Running AI at the edge can feel like juggling a dozen balls simultaneously – different vendors, platforms, and complex regional requirements. Managing this ecosystem of diverse edge devices, software frameworks, and networking components adds maintenance, security, and compliance costs.
- Data movement and storage: Transferring large volumes of data between edge devices and centralized cloud infrastructure can lead to significant network and storage expenses.
- Energy consumption: AI inference at the edge can be power-intensive, increasing operational costs, especially in remote or resource-constrained environments.
Optimizing costs without sacrificing performance
To make edge AI financially viable, businesses must leverage strategies that balance efficiency and cost-effectiveness. Key approaches include silicon diversity, serverless inference, and real-time data integration.
Leveraging silicon diversity
One of the most innovative ways to optimize costs at the edge is by matching the right compute to each task. Instead of defaulting to the most expensive AI accelerators, businesses can optimize performance with diverse silicon architectures tailored to specific workloads. That requires silicon diversity – access to different types of specialized chips designed for specific AI workloads.
With demand for AI-optimized chips outpacing supply, enterprises can adopt a mix of CPUs and GPUs to right-size performance, control costs, and scale efficiently across global edge locations.
Embracing serverless inference
Traditional AI inference models require dedicated infrastructure, which can be costly and inefficient. Serverless inference allows enterprises to scale AI workloads dynamically, only paying for their computing power rather than overbuying hardware or scrambling to upgrade with every AI innovation.
It also takes a big load off your team. Instead of worrying about managing infrastructure, they can focus on building better AI models. Plus, serverless gets AI-powered applications up and running faster, so you can keep pace with business needs.
Localizing real-time data integration
Running inference at the edge helps organizations avoid unnecessary data transfer costs and reduce the risk of compliance violations. By processing sensitive data locally, businesses can maintain tighter control, meet data residency requirements, and sidestep the steep penalties of mishandling regulated information. It also allows organizations to fine-tune AI models using local data for more accurate and relevant insights.
Technologies like Retrieval-Augmented Generation (RAG) and managed data streaming platforms like Kafka help make this possible. With vector stores and real-time pipelines, models can securely access proprietary data, public sources, and even synthetic datasets without transferring data across regions or retraining from scratch.
Building a better edge
A successful edge AI strategy goes beyond choosing diverse hardware – the software and infrastructure layers also impact cost and performance and are equally important. Selecting AI frameworks and runtime environments optimized for edge deployment minimizes resource consumption and improves performance. Similarly, if you want to scale AI cost-effectively, you need a flexible, open, and composable infrastructure that gives you the freedom to choose the hardware, models, and software that fit your needs. Partner with providers that offer scalable and geographically distributed edge infrastructure, ensuring that you only pay for what you need while minimizing latency.
This composable AI stack makes integrating the best tools at every layer across infrastructure, data, and applications easier. It also helps future-proof your strategy. As new technologies emerge, you can evolve quickly without being locked into a single vendor or platform.
The future of edge AI: Intelligent, affordable and scalable
Organizations scaling edge AI successfully aren’t necessarily spending more, but they are spending smarter. Success lies in striking a balance between high performance and cost efficiency. By understanding cost drivers and embracing the right infrastructure, organizations can maximize the benefits of edge AI without overspending.
About the author
Kevin Cochrane, chief marketing officer, Vultr is a 25+ year pioneer of the digital experience space. He is now working to build Vultr’s global brand presence as a leader in the independent cloud platform market.
BrainChip and ISL advance AI-powered radar for military and aerospace
Article Topics
AI/ML | digital infrastructure | edge AI | edge cloud | edge computing | GPU | Vultr
Comments