BrainChip says new standards needed for edge AI benchmarking
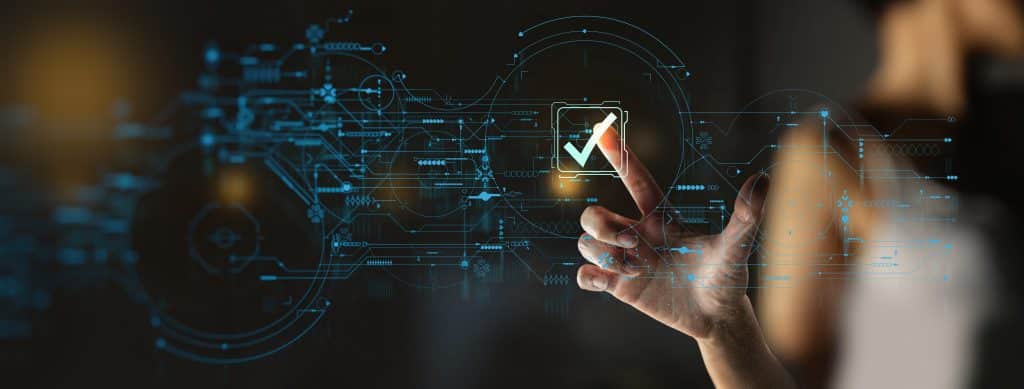
BrainChip, a provider of neuromorphic processors for edge AI on-chip processing, has published a white paper that examines the limitations of conventional AI performance benchmarks. The white paper also suggests additional metrics to consider when evaluating AI applications’ overall performance and efficiency in multi-modal edge environments.
The white paper, “Benchmarking AI inference at the edge: Measuring performance and efficiency for real-world deployments”, examines how neuromorphic technology can help reduce latency and power consumption while amplifying throughput. According to research cited by BrainChip, the benchmarking used to measure AI performance in today’s industry tends to focus heavily on TOPS metrics, which do not accurately depict real-world applications.
“While there’s been a good start, current methods of benchmarking for edge AI don’t accurately account for the factors that affect devices in industries such as automotive, smart homes and Industry 4.0,” said Anil Mankar, the chief development officer of BrainChip.
Recommended reading: Edge Impulse, BrainChip partner to accelerate edge AI development
Limitations of traditional edge AI benchmarking techniques
MLPerf is recognized as the benchmark system for measuring the performance and capabilities of AI workloads and inferences. While other organizations seek to add new standards for AI evaluations, they still use TOPS metrics. Unfortunately, these metrics fail to prove proper power consumption and performance in a real-world setting.
BrainChip proposes that future benchmarking of AI edge performance should include application-based parameters. Additionally, it should emulate sensor inputs to provide a more realistic and complete view of performance and power efficiency.
“We believe that as a community, we should evolve benchmarks to continuously incorporate factors such as on-chip, in-memory computation, and model sizes to complement the latency and power metrics that are measured today,” Mankar added.
Recommended reading: BrainChip, Prophesee to deliver “neuromorphic” event-based vision systems for OEMs
Benchmarks in action: Measuring throughput and power consumption
BrainChip promotes a shift towards using application-specific parameters to measure AI inference capabilities. The new standard should use open-loop and closed-loop datasets to measure raw performance in real-world applications, such as throughput and power consumption. BrainChip believes businesses can leverage this data to optimize AI algorithms with performance and efficiency for various industries, including automotive, smart homes and Industry 4.0.
Evaluating AI performance for automotive applications can be difficult due to the complexity of dynamic situations. One can create more responsive in-cabin systems by incorporating keyword spotting and image detection into benchmarking measures. On the other hand, when evaluating AI in smart home devices, one should prioritize measuring performance and accuracy for keyword spotting, object detection and visual wake words.
“Targeted Industry 4.0 inference benchmarks focused on balancing efficiency and power will enable system designers to architect a new generation of energy-efficient robots that optimally process data-heavy input from multiple sensors,” BrainChip explained.
BrainChip emphasizes the need for more effort to incorporate additional parameters in a comprehensive benchmarking system. The company suggests creating new benchmarks for AI interference performance that measure efficiency by evaluating factors such as latency, power and in-memory and (on-chip) computation.
DartPoints introduces new bare metal cloud solution
Article Topics
AI/ML | benchmarking | Brainchip | chip | industry standards | MLPerf | performance
Comments