AI for enterprise growth: A strategic guide to data management and monitoring
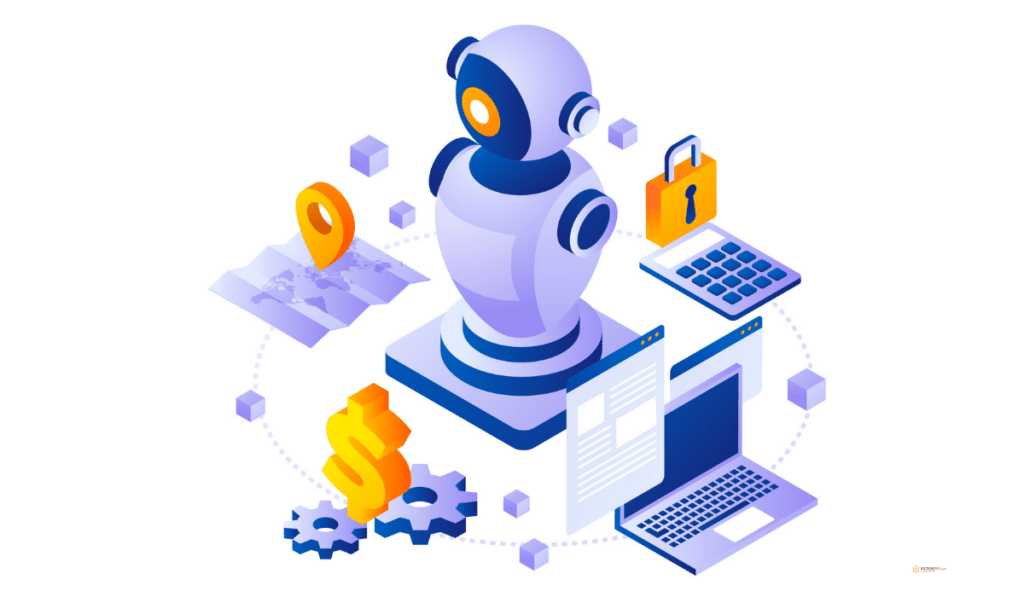
By Sawaram Suthar, founding Director at Middleware
The enterprise AI market is projected to grow from $38.19 billion in 2024 to an impressive $311.64 billion by 2029, with an annual growth rate of 52.17%. This rapid expansion is largely driven by the increasing use of AI in data management and monitoring, which is transforming the way businesses operate.
With AI’s help, you can ensure that your business operations are more efficient, leading to reduced costs and assured business growth and competitiveness. You can make better decisions and easily solve complex problems. This will improve customer experience and engagement with your organization.
This article will explain how businesses can use AI to drive growth and integrate it effectively.
Understanding AI’s role in enterprise data management
Mentioned below are some strategies to transform your enterprise data management with the help of AI.
The AI advantage
- Accelerated data processing: AI improves your organization’s data processing capabilities by automating repetitive tasks, leading to greater efficiency and fewer errors. This automation allows your team to focus on more strategic initiatives.
- Optimized decision-making: By analyzing historical data and current trends, AI accurately predicts future scenarios. These insights refine your business strategy and address challenges. Additionally, AI algorithms continuously evolve and remain unbiased, resulting in more effective decision-making.
- Scalability and adaptability: Integration of AI in enterprise data management is all the more effective because it can scale with your organization’s needs and data size. AI also ensures more efficient big data management by rapidly identifying data types, finding possible connections among the datasets quicker, and drawing meaningful conclusions from them.
Integration with infrastructure monitoring
- Real-time monitoring: Using AI for real-time infrastructure monitoring gives useful insights for better and faster performance with effective resource use. AI supports predictive maintenance, enhances security, reduces costs, and improves scalability.
- Best practices: The best practices for effective AI and monitoring integration are implementing data automation, managing disparate sources, enabling data monitoring, promoting no-code integrations, using data connectors, ensuring data privacy and data security, and adopting a scalable data fabric.
Challenges in AI adoption
While AI provides an edge in this competitive and dynamic business world, enterprises need to overcome certain challenges to adopt AI-based systems successfully.
Addressing data privacy and security
- Regulatory compliance: When adopting AI models, companies need to comply with data privacy regulations such as GDPR and CCPA. AI tools should collect only necessary data and inform individuals about what is collected and its purpose to prevent misuse.
- Security risks: One more hurdle in adopting AI is recognizing and addressing security risks tied to AI-driven data management. Companies need regular threat modeling and security assessments throughout the development process to protect AI algorithms against attacks and unauthorized changes.
Overcoming integration hurdles
- Legacy system integration: Another challenge in AI adoption is integrating with legacy systems, most often due to incompatible data formats, limited API capabilities, and outdated architectures. Invest time and resources to ensure compatibility and performance post-integration.
- Cost management: AI systems can be expensive and, without proper management, can lead to resource wastage. Scalable systems that allow regular assessments to align these systems with the company’s expectations are crucial for managing expenses.
Future outlook
AI is a vast and constantly evolving technological landscape that, with the current rate of improvements in machine learning and natural language processing, can take on several time-consuming tasks. Here’s a look at the future of AI use in business.
Emerging trends and technologies
- Effective data implementation: Businesses should ensure they’re using data tracing technologies effectively by creating clear processes, following industry guidelines, and regularly checking and improving their systems. Big data and AI can also provide solutions that grow with the business and offer smart insights for better decisions.
- AI innovations: Some popular AI-related innovations to watch out for to stay ahead in the changing business landscape are conversational AI, predictive analytics, low-code and no-code AI, ethical and explainable AI, multi-modal AI, digital twins in the context of Industry 4.0 and IoT, and edge computing.
- Technological integration: For technological integrations, you need smooth integration of current systems with new technologies while ensuring they meet industry regulations. The integration must be scalable and adaptable to future technological advancements while maintaining consistent, reliable performance over time.
Strategic advice for enterprises
- effective implementation of AI. This strategy helps you answer essential questions like what AI can do for your business.
- To effectively navigate the evolving landscape of AI and monitoring technologies, align your AI strategies with your business objectives and assemble a team of experts to guide and drive your organization through AI innovations. Build a robust data infrastructure and implement continuous monitoring and adaptation.
Conclusion
Integrating AI for organizational data management and monitoring improves the efficiency and accuracy of your operations and makes it easier for your data to scale.
AI adoption speeds up data processing while also giving access to real-time insights. This supports predictive maintenance, ensures optimized performances, and reduces costs.
Implementing AI for enterprise growth offers numerous opportunities for success. Your organization will get a faster and more secure system that focuses on a strategic approach to effective data management.
About the author
Sawaram Suthar (Sam) is a Founding Director at Middleware. He has extensive experience in marketing, team building, and operations. He is often seen working on various GTM practices and implementing the best ones to generate more demand.
BrainChip advances edge AI with ultra-low power processing
Article Topics
AI adoption | AI/ML | data management | generative AI | Middleware | monitoring
Comments