AI driving demand for inference computing needs
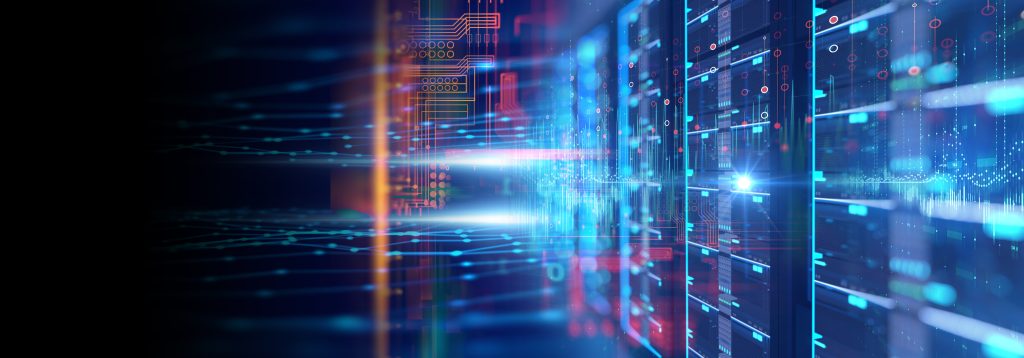
By Tony Grayson, General Manager, Compass Quantum
The evolution of edge or modular data centers has yet to meet initial expectations, largely due to insufficient network infrastructure and the lack of a commercially viable platform that requires local computing. Despite this, there is a growing shift toward modular solutions that adhere to hyperscale standards, a trend being adopted by enterprises, the Department of Defense, and various federal and state agencies.
This shift is driven by several factors, including but not limited to the rapid advancement of technology, the increasing need for a shorter time to market, the complex requirements for AI power and cooling, sustainability goals, data sovereignty and local power limitations.
For example, the GB200, Nvidia’s next super chip, requires a direct-to-chip liquid solution because it will be approximately 132kW per rack and has 30x the performance increase in inference AI. With the increased performance of the new chipset, an 8000 GPU, 15MW data center will now only need 2000 GPUs and use 4MW. The trend is as power density increases along with performance, the overall number of racks and power goes down. So if you are a business, what do you design your data center for? This generation? The next generation? Each has significant capital considerations.
While the trend towards modular data centers is increasing it still remains under the radar, overshadowed by the industry’s focus on AI and the growth of large hyperscale data center campuses.
In my first of three columns, I’m drilling down on how artificial intelligence and the technology needed to support it will influence critical infrastructure decisions when it comes to edge computing deployment in our current market – and it all starts with inference AI.
Inference refers to the process where a trained model uses learned information to make predictions or decisions based on new, unseen data.
In generative AI, inference generally refers to generating new data instances after the model has been trained. Training involves learning a dataset’s patterns, features, and distributions. Once the training is complete, the model uses this learned information to generate new content that resembles the original data but is uniquely generated. When text based, this is most likely not latency sensitive but could become more latency sensitive with more “rich” data like video files.
In more traditional uses of AI, inference refers to applying a trained model to new data to make predictions or classifications. This is common in models used for tasks like image recognition, natural language processing (excluding generation), or any other form of decision-making based on learned data patterns. This is often latency sensitive because the model needs to make quick decisions.
Inference AI is being employed in various sectors, with impacts on safety, quality control, network technology, and emergency response.
In the realm of safety, a notable example is the partnership between T-Mobile and Las Vegas for pedestrian safety. This initiative aims to reduce pedestrian fatalities at high-traffic crosswalks. The AI system involved checks the status of traffic lights when a pedestrian enters a crosswalk. If the light is not red, the system rapidly assesses approaching traffic and can change the light to red within milliseconds if there is a risk of a collision.
Quality control in manufacturing has also benefited greatly from AI. AI models are essential for identifying product defects by analyzing images from assembly lines. These models can instantly detect anomalies or defects, processing vast amounts of visual data in microseconds. This capability allows for immediate corrections, reducing waste and enhancing the efficiency of manufacturing processes.
In the telecommunications sector, advancements in 5G and upcoming 6G Radio Access Network (RAN) technology are poised to revolutionize industries such as autonomous driving and real-time virtual reality experiences. These applications demand ultra-low end-to-end latency to match or exceed human reaction times, far beyond the capabilities of traditional cloud computing infrastructures. The ultra-low latency is particularly critical in autonomous vehicle operations, where the swift delivery of data packets and rapid inference processing are vital for ensuring safety and optimizing performance.
AI-driven emergency response systems are another critical application of inference AI. These systems are designed to detect and respond to natural disasters through immediate data analysis, enabling prompt alerts and coordinated responses. For instance, an AI system can analyze seismic data to identify earthquake patterns and instantly trigger emergency alerts in affected areas. This real-time processing capability can provide crucial extra time for people to take protective actions, potentially saving lives. The effectiveness of such systems hinges on their ability to process data and make predictions almost instantaneously.
The question is though, with vacancy at local data centers at an all-time low, where will you place your racks to support the inference platform you are working on? The good news is there’s a solution that addresses this and growth in hybrid and multi-cloud computing, support for higher-density racks and the relentless increase in the global volume of data.
That solution is Quantum. Modular data centers make it possible to rapidly deploy IT capacity whenever and wherever needed. Rooftops, parking lots, fields – no problem! Perhaps best of all, the rack-ready structure that supports AI inference can be deployed and running in months rather than years—a critical differentiator when there is such a backlog for the construction of data center facilities.
Compass Quantum provides an efficient design and can support very high-power-density per rack. Quantum is also site-agnostic, giving customers the flexibility to locate extra capacity next to their existing hyperscale centers where power and fiber already exist. Speed and scalability for future AI needs gives customers what they need with near-term benefits that don’t rely on hyperscale capacity.
In the face of sweeping changes across the infrastructure and networking landscape, edge deployments serve current and future technological landscapes perfectly. The pace of digital transformation, compounded by the growing demand for AI, high-performance computing and equitable broadband access, emphasizes the critical need for agility and rapid deployment of computing resources. Our flexible, scalable and efficient Quantum solution delivers quickly against the urgent requirements of AI-driven edge computing solutions.
About the author
Tony Grayson leads Compass Quantum, a division of Compass Datacenters dedicated to delivering turnkey, modular data centers and giving customers the flexibility to remotely monitor, manage, and operate these locations. Before joining Compass, Tony was an SVP at Oracle, where he was responsible for their physical infrastructure and cloud regions. He has also held senior positions with AWS and Facebook. Before embarking on his data center career, Tony served for 20 years in the United States Navy.
DISCLAIMER: Guest posts are submitted content. The views expressed in this post are that of the author, and don’t necessarily reflect the views of Edge Industry Review (EdgeIR.com).
The role of edge computing in expanding cloud AI deployments
Article Topics
AI | Compass Datacenters | edge computing | inference computing
Comments