Adapting datacenter compute power to the Edge
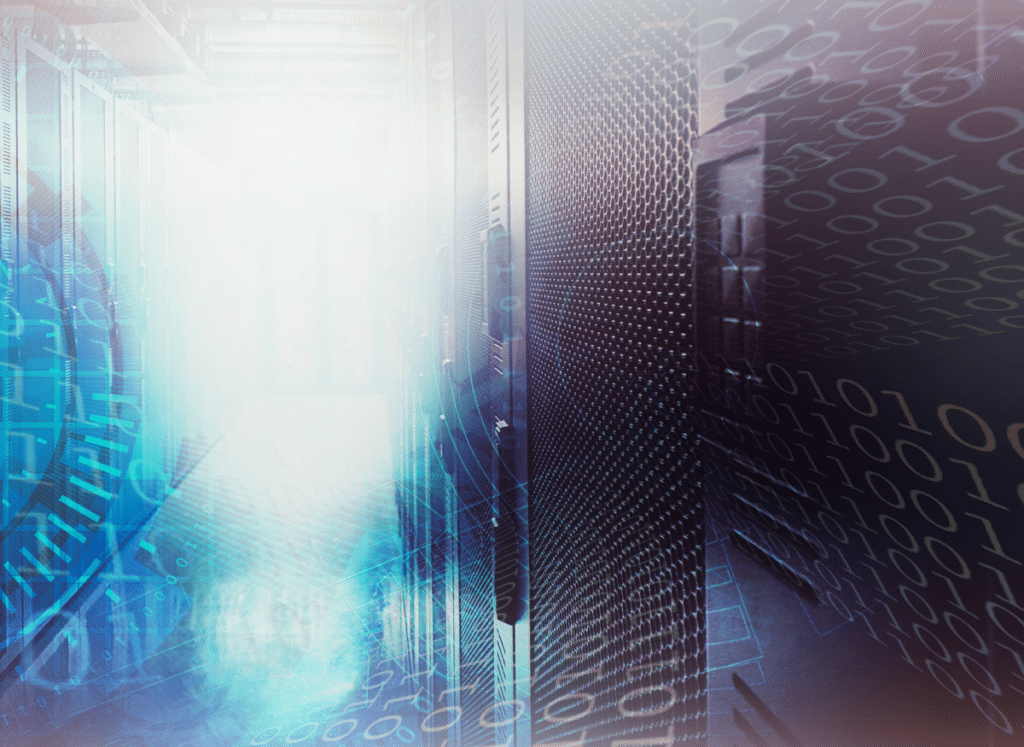
By Braden Cooper, Program Manager at One Stop Systems, Inc.
Every computing and data processing industry is facing the same challenge: the size of data is growing beyond the capabilities of existing computing infrastructure. The surge in data is in turn leading to rapid growth in storage, computing, and networking gear which process and distribute the data. At the same time, the development of AI applications across industries has also generated demand for higher and higher compute power at the location the data is captured—the edge, in tech parlance.
Datacenters, with no significant size, weight, or power restrictions, have a simple task in adapting—either scale out with additional racks or replace the outdated hardware with newer systems.
Edge environments, however, face an entirely different challenge (and opportunity) in bringing the new technologies and AI applications to the environments where the data is captured.
Object classification, pattern detection, pathfinding, and other AI use cases have changed the compute capacity requirements of edge applications. While edge systems have typically achieved the size, weight, and power restrictions of their respective applications through lower compute capacity embedded components, the new landscape of edge computing is calling for datacenter level compute capacity in a shape optimized for the edge. In addition, to implement a real-time AI image recognition inferencing system in applications such as self-driving cars, the criticality and time-sensitivity of the inferencing results prohibit a datacenter-based cloud upload, process, download, and communicate cycle. The computing must happen at the location in which the data is captured, and the compute capacity must be sufficient to meet the real-time demands of the application.
Designing edge compute systems for AI
To run an AI application, the edge compute server must have data ingest, storage, compute, and networking. For real-time applications, these building blocks should be load-balanced so that the data can flow from ingest to action without being bottlenecked at any one point. In defining the hardware architecture, the compute capacity is often the primary bottleneck due to the high power and size requirements of high-end AI compute GPUs. This restriction in turn leads to limited sensor ingest and an ultimately compromised inferencing application that does not deliver the level of information needed.
To circumvent these compromises in the edge AI workflow, the edge system architect must look to adapt the power of datacenter computing to the edge environments.
Adapting datacenter compute capabilities to edge environments are the same challenges designers of edge computing systems have always faced: size, weight, power, vibration, and humidity. For size and weight, datacenter AI computing systems are often inefficiently designed in a steel construction rackmount enclosure of a fixed height and length without consideration for the precious allocation of these attributes in edge environments. The same system can often be optimized for edge environments by making the enclosure narrower in all dimensions and ruggedized to support the components within.
Materials options such as aluminum, stainless steel, or carbon fiber allow for a reduction in weight and improved stiffness to weight ratios.
In optimizing the enclosure space, the mechanical design can provide more efficient cooling, more selective weight distribution and reduction and add support structures to meet shock and vibration requirements. The mechanical design for the optimized package can be validated using computational fluid dynamics (CFD) and finite element analysis (FEA) simulation packages, then tested and qualified to the rigid environmental requirements of the edge environment. For environments with humidity or dust-related requirements, the system components can be conformal coated, or replaceable air filters added to the design.
As the size of data continues to scale alongside the applications of AI across many different industries, edge architectures must avoid compromise on compute capacity to keep up. Adapting datacenter compute technology in edge spaces is not without challenges, but edge computing designers know how to handle these challenges. Edge optimized AI compute appliances allow a clear path to a scalable infrastructure to meet the needs of the always-expanding data in need of computing.
About the author
Braden Cooper is Program Manager at One Stop Systems, Inc., a provider of high-performance edge computing systems.
DISCLAIMER: Guest posts are submitted content. The views expressed in this blog are that of the author, and don’t necessarily reflect the views of Edge Industry Review (EdgeIR.com).
Capgemini and Viavi enter new 5G and edge-focused collaboration
Article Topics
datacenter | edge AI | edge server | One Stop Systems | ruggedized | transportable
Comments